The only constant in life is change - Heraclitus
Continuous learning and knowledge sharing have been critical drivers of human progress. The rise of the internet has elevated these activities to unprecedented levels, inundating us with vast amounts of data and information. In such an environment, how can one successfully find structured information to drive progress effectively? This challenge is especially relevant when using Large Language Models (LLMs). How can users and applications best leverage LLMs, and what kinds of value can they hope to derive from them?
The utilization of GenAI applications, particularly chatbot implementations, is growing exponentially. This also opens up new opportunities for effective skill development by smartly leveraging available data. For instance, if half of your team is constantly asking the chatbot how to optimize the data pipeline process, it provides valuable insights into where there are gaps in documentation and knowledge. But how can this information be automatically retrieved and transformed into actionable recommendations?
Databricks Inference Tables: The Basis for Strategic Learning
Before you can begin planning learning initiatives, you naturally need to know where to focus. Identifying the biggest gaps in knowledge and skills is crucial. For this purpose, Mosaic AI's out-of-the-box inference tables are unparalleled. They automatically log user prompts, allowing you to see the types of information that are consistently sought after. For more detailed information about Databricks Mosaic AI and inference tables, please visit the previous article here: Simplifying GenAI Architecture with Databricks Mosaic AI Gateway (ikidata.fi)
Inference tables play a crucial role in monitoring and diagnosing AI and ML models in production workflows. By continuously logging serving request inputs and their corresponding predictions from Mosaic AI Model Serving endpoints into a Delta table in Unity Catalog, these tables simplify the tracking process. This integration allows you to leverage the full capabilities of the Databricks platform, including SQL queries, notebooks, and Lakehouse Monitoring, to effectively monitor, debug, and optimize your models. Inference tables can be enabled on any existing or new model serving endpoint, ensuring that all requests to the endpoint are automatically logged into the table in Unity Catalog.
Now that we have activated inference tables and are capturing the usage data of LLM models, what’s next?
Creating an Automated Feedback Loop to Optimize Organizational Learning
First, we need to build a robust solution architecture to clearly describe the entire process.

User prompts are generated when a user or AI agent interacts with LLM models through applications or notebooks.
All traffic is routed through Mosaic AI Model Serving, and requests are logged into inference tables.
Another GenAI application categorizes all user prompts with short summaries based on predefined categories, using Mosaic AI Function Calling.
GenAI summarizes the situation using all available short summaries and proposes appropriate actions and learning suggestions.
Continuous monitoring is conducted using AI/BI dashboards.
From words to actions: organizing learning sessions and improving documentation based on real data.
Simplifying monitoring with AI/BI dashboards
Once the process is automated, you can focus on analyzing the results and implementing the action points.
This demonstration shows how you can easily and effortlessly start utilizing Inference Tables to enhance learning activities in your organization. The Databricks Data Intelligence Platform provides all the necessary components to automate the entire process—although automating the learning itself isn't possible (yet?). Nonetheless, the opportunities are truly fascinating!
Ikidata is a pioneer in GenAI Agent automation, providing deep insights into this emerging technology from technical, architectural, and business perspectives. We make it simple to bring your ideas to life.
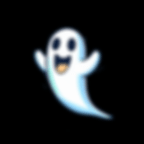
-𝐾𝑟𝑎𝑡𝑡𝑖
Databricks Excellency Services