For the past year, Generative AI (GenAI) has captured everyone’s attention and sparked countless conversations. Yet as buzzwords and acronyms multiply, staying fluent in the latest terminology can feel overwhelming. To help you cut through the noise, we’ve created concise summaries of the key concepts you need to know. Think of this as your go-to GenAI guide—quick, clear, and right at your fingertips. It’s time to dive into GenAI terms one by one and get fluent in GenAI.

AI, short for "Artificial Intelligence," is an umbrella term describing computers and machines capable of performing tasks that typically require human intelligence. It encompasses various types and approaches, each designed to tackle different challenges and functionalities.

GenAI
GenAI stands for "Generative Artificial Intelligence." It's like having a super-smart friend who has read every book, seen every picture, and listened to every song. This friend can create new stories, images, music, and more, based on everything they've learned.
GenAI is usually a Large Language Model (LLM). This means it's trained on a vast amount of text and data from the internet, books, articles, and more. Because of this extensive training, it can understand and generate human-like text and other content.
For example, if you want a unique recipe, GenAI can come up with one by combining ingredients and cooking methods it has learned from countless recipes. Or, if you need a new marketing slogan, GenAI can generate creative suggestions by drawing from all the ads and slogans it has seen.
In short, GenAI uses a vast amount of data to generate new and original content, making it a powerful tool for creativity and problem-solving.

Large Language Model (LLM)
Think of large language models (LLMs) as incredibly knowledgeable and sophisticated AI assistants that can understand and generate human-like text. These models have been trained on vast amounts of data, enabling them to perform a wide range of tasks, from answering questions to creating content. Some of the most popular LLMs are provided by OpenAI (ChatGPT), Meta (LLaMA), and Anthropic (Claude). These models are evolving rapidly, and new players are continually emerging, pushing the boundaries of what AI can achieve.

Machine Learning
Although GenAI is currently the coolest kid in town, it's vital to understand machine learning.
Machine learning is a subset of AI that focuses on algorithms and models that enable computers to learn patterns and make predictions from data without explicit programming. ML algorithms can be trained on labeled data to recognize patterns and make decisions or predictions based on new, unseen data.
Imagine you have a bunch of emails and you want to sort them into "spam" and "not spam." You feed a machine learning algorithm lots of examples of both spam and not spam emails. The algorithm learns from these examples and then can predict whether new emails are spam or not based on what it has learned. It's like teaching a friend how to recognize a genre of movies by showing them many examples; soon they can predict if a new movie fits that genre.
There are different types of machine learning: supervised, unsupervised, and reinforcement learning.
Supervised Learning: This is like a teacher-student relationship. You provide the algorithm with labeled examples (like spam and not spam emails), and it learns to make predictions based on this labeled data.
Unsupervised Learning: Here, the algorithm is left to discover patterns and relationships in the data on its own, without any labeled examples. It's like giving your friend a collection of movies without telling them the genres and asking them to group similar ones together.
Reinforcement Learning: This is akin to training a pet with rewards and punishments. The algorithm learns by interacting with an environment and receiving feedback in the form of rewards or penalties. Over time, it learns the best actions to take to maximize its rewards.

Deep Learning
Deep learning is a subset of AI that involves the use of artificial neural networks, inspired by the structure and functioning of the human brain. Deep learning models, particularly deep neural networks, consist of multiple layers of interconnected neurons that learn hierarchical representations of data. They excel at learning complex patterns and have achieved remarkable success in various domains, including image and speech recognition.
Think of deep learning like teaching a child to recognize animals. You show the child thousands of pictures of dogs and cats. Over time, the child learns to identify the subtle differences between the two. Similarly, a deep learning model can learn to recognize objects in images by analyzing millions of examples and identifying patterns, like distinguishing a dog from a cat. It's akin to showing someone countless movie scenes until they can tell which director's style it is or which actor is performing, based on the intricate patterns and styles they've observed.
Both machine learning and deep learning are powerful tools that help in making predictions, recognizing patterns, and making sense of large amounts of data. They form the backbone of many technologies we use today, from email filters to image recognition systems.

Hallucination
Imagine a large language model (LLM) like a super-smart storyteller. You ask it a question, and it tries its best to give you an answer based on all the stories, books, and information it's read.
But sometimes, this storyteller gets a bit too creative. Instead of saying, "I don't know," it might make up an answer that sounds really convincing but isn't actually true. It's like asking your friend for movie recommendations, and they confidently tell you about a fantastic film that doesn't even exist because they got mixed up. This creative mix-up is called a "hallucination" in LLM models. The model isn't trying to trick you; it's just trying too hard to be helpful and ends up imagining things that aren't real!

RAG (Retrieval-Augmented Generation)
Now, let's give our storyteller a magical cinema pass! With this pass, whenever you ask a question, the storyteller can quickly dash off to a gigantic movie archive filled with real films, reviews, and trustworthy information. The storyteller watches a bit of the movie, reads the reviews, and then comes back to you with a well-informed recommendation.
This magical process is called Retrieval-Augmented Generation (RAG). It helps our storyteller stay grounded in reality by checking its facts in the big archive before giving you an answer. So, you get the best of both worlds: the creativity of the storyteller and the accuracy of the movie archive!
From a technical perspective, it's essentially prompt enrichment. Contextual data is retrieved from a RAG dataset, and the most relevant information is appended to your prompt when using the LLM.

LLM-as-a-Judge
Even judges aren't safe from GenAI development! Now it's possible to use LLM as an evaluator instead of doing that manual human work. LLM-as-a-Judge acts as a brilliant AI evaluator in a writing competition for other AI systems! This judge utilizes specific guidelines (evaluation prompts) to assess the texts generated by chatbots, Q&A systems, or any text-producing AI. It evaluates clarity, coherence, and adherence to the rules, much like a talent show judge rating performances.

GenAI Agents
Think of GenAI Agents like having a team of super-smart robots, each with their own special skills, working together to help you out. Imagine you have different tasks: one robot can write stories, another can answer trivia questions, and another can help you plan your day. You give them instructions, and they all collaborate to get things done efficiently.
For example, you might say, "Hey team, I need a funny story about a talking cat, some interesting facts about space, a movie recommendation, and a schedule for my weekend." Each robot jumps into action—one writes a hilarious cat story, another pulls up cool space facts, another suggests a great film to watch, and another organizes your weekend plans. GenAI Agents are like this team of specialized robots. They work together, using their individual strengths to help you with a wide range of tasks, making your life easier and more fun!
Hopefully, with these explanations, you'll gain a better grasp of the meanings behind the terminologies in the world of GenAI. The field is evolving rapidly and can seem quite technical at first. While these terms may sometimes sound like gibberish, here's a secret: the underlying reality is actually much simpler.
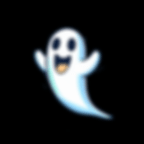
-𝐾𝑟𝑎𝑡𝑡𝑖